Results for ""
Nasscom Responsible AI Resource Kit
The Responsible AI Resource Kit is the culmination of a joint collaboration between Nasscom and leading industry partners to seed the adoption of responsible AI at scale.
The Resource Kit comprises sector-agnostic tools and guidance to enable businesses to leverage AI to grow and scale with confidence by prioritising user trust and safety.
To retain and improve the Kitʼs utility over time, Nasscom intends to maintain it as an evolving reference, progressively building on the latest research and best practices for responsible AI adoption generated by committed stakeholders from the industry, government, academia, think tanks, and civil society organisations, while recognising that responsible AI governance must lean on the precepts of multi-stakeholderism.
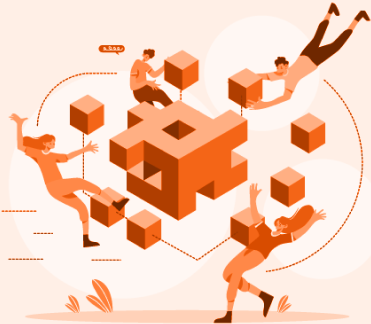
Responsible AI Principles

Inclusivity & Non-Discrimination
AI systems must be fair and inclusive, not fostering prejudices, discrimination or preference for an individual, a community or a group based on their sensitive attributes (e.g., race, gender, ethnicity).

Reliability & Safety
AI systems must produce consistent and reliable outputs in all scenarios. Appropriate grievance redressal mechanisms should be put in place to address cases of adverse impact.

Privacy
AI systems should respect user privacy. Users’ right to know what data is collected, why it is collected and who has access to it should be protected. AI systems should not use the data for purposes other than what is stated.

Security
AI system should be robust and secured against adversarial attacks and malicious use. Identifying and mitigating system vulnerabilities is critical.

Transparency
AI systems should be transparent about how they were developed, their processes, capabilities and limitations, to the extent possible.

Explainability
AI systems should be explainable to users significantly impacted by their decisions. Explanations must be provided free of cost in non-technical, intuitive language.

Accountability
Organisational structures and policies should be created to clarify who is accountable for the outcomes of AI systems. Human supervisory control of AI systems is recommended.

Protection and Reinforcement of Positive Human Values
AI systems should be designed and operated such that they align with human values. AI should promote positive human values for the progress of humanity as a whole.

Compliance
Throughout their lifecycle, AI systems should comply with all applicable laws, statutory standards, rules, and regulations – Organizations should be watchful of the evolving AI regulatory landscape and ensure compliance at all times.
Responsible AI Principles in Practice
Responsible AI Maturity Assessment
Enabling enterprises to independently assess and monitor the development and deployment of AI solutions for ethical compliance
Take the Assessment
Governance Framework
Recommending management tools and structures for enterprises to assess and mitigate ethical risks arising from the deployment of AI solutions
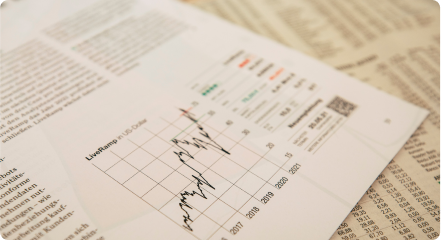
Risk Identification and Assessment Tool
Enabling systematic monitoring and assessment of potential risks arising from enterprise AI adoption
Learn more
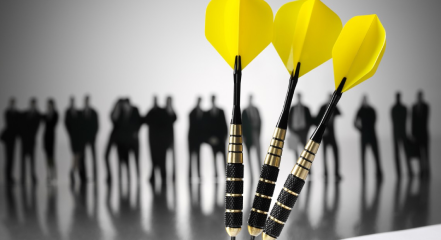
Principles and Points of Focus
Recommending points of focus for enterprises to implement responsible AI principles and mitigate ethical risks arising from AI adoption
Learn more
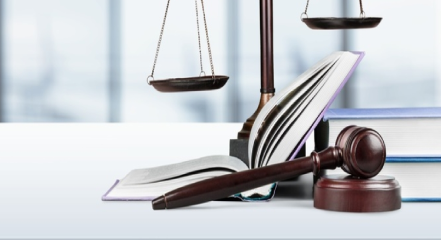
Ethics Committees
Proposing models in which ethics committees may be configured to maintain internal oversight of the development and deployment of enterprise AI solutions
Learn more
Architect’s Guide
Prescribing responsible AI best practices implementation methods and tools for enterprise adoption
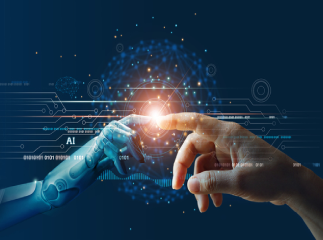
Responsible AI Lifecycle
Illustrating adherence to responsible AI principles at each stage of the AI project value chain
Learn more
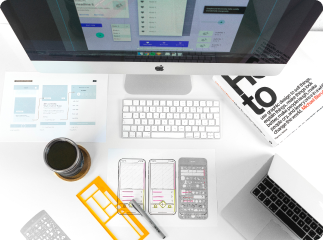
Understanding Human-Centered Design
Recommending guidelines for the adoption of a human-centered approach to building AI systems
Learn more
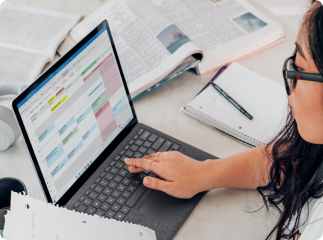
Envisioning and Impact Assessment
Providing high-level guidelines and recommendations for impact assessment of a system in its early stages
Learn more
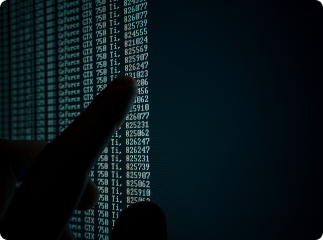
Data Collection & Processing
Providing a primer on data bias and its common types, and proposing recommendations and best practices for responsible data collection and processing
Learn more
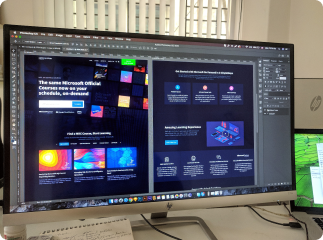
Prototyping
Providing primer on processes and tools to prototype a system for responsible release
Learn more
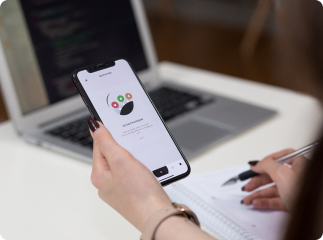
Testing
Recommending methods and techniques for model testing to ensure compliance with responsible AI principles
Learn more
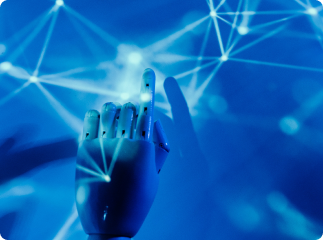
Building AI for Production
Providing insights into challenges around model development with DevOps and recommending responsible ML toolkits as a superior alternative
Learn more
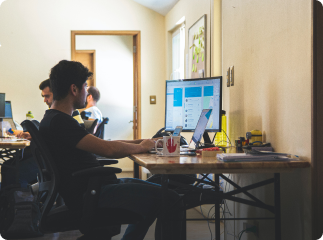
Deployment
Recommending tools and best practices for responsible deployment of the system
Learn more
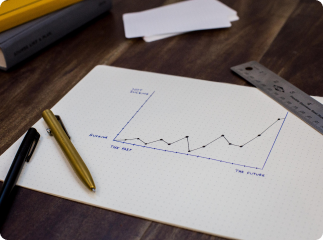
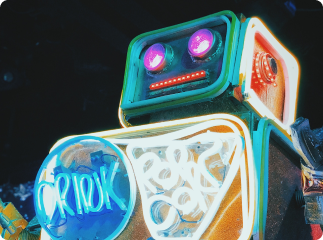
Tools for Responsible AI
Discussing strategies and tools to mitigate privacy and security risks
Learn more
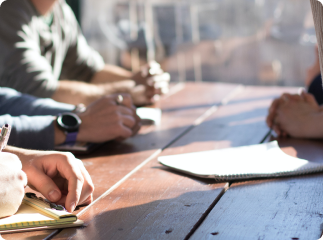
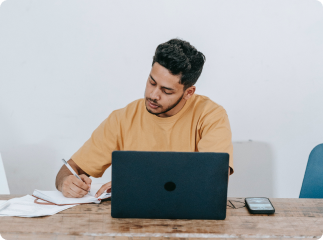
OUR CONTRIBUTORS
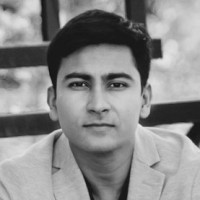
Akbar Mohammed,
Architect, Fractal Analytics
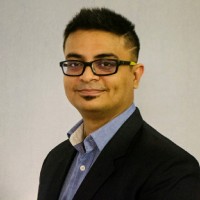
Akshat Chaudhari,
Customer Service Support Manager, Big Data, Microsoft
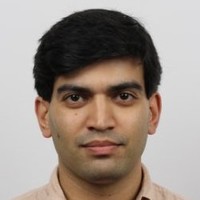
Amit Deshpande
Researcher, Microsoft Research India
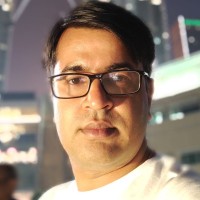
Amit Kumar
Associate Director, Data Science and Machine Learning, Deloitte India
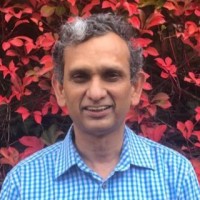
Anantha Sekar
Product Management Group, Experience and Intelligence, Tata Consultancy Services
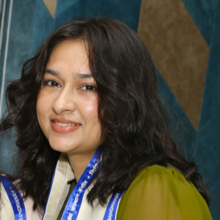
Anjali Pathak
Product & Social Media Lead (INDIAai), Nasscom
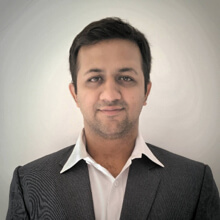
Ankit Bose
Head of AI, Nasscom
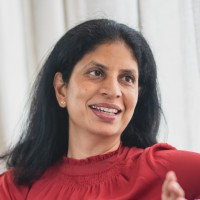
Aparna Gupta
Executive Director, Customer Success, Microsoft
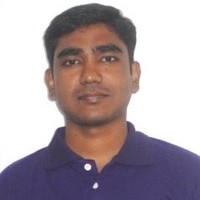
Balaji Ganesan
Senior Research Engineer, IBM Research
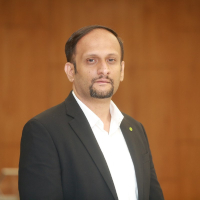
David George
Director, Risk Advisory, Deloitte India
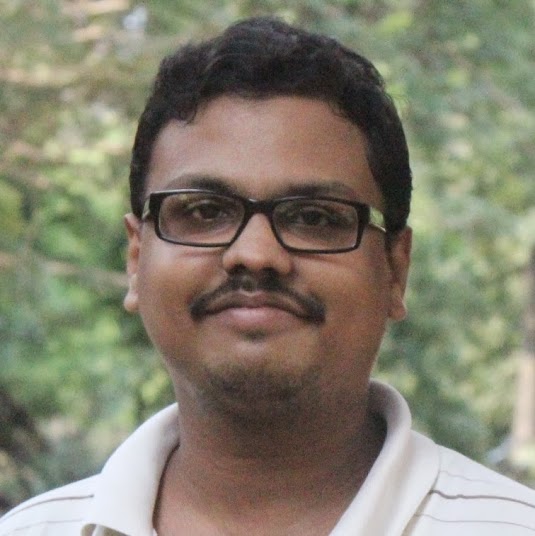
Deepak Vijaykeerthy
Research Engineer, Data and AI, IBM Research
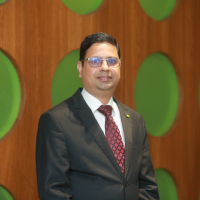
Johar Batterywala
Partner, Deloitte Haskins & Sells
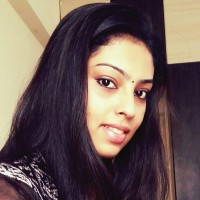
Keerthana Kennedy
Assistant Consultant, Tata Consultancy Services
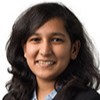
Krutika Choudhary
Consultant, Fractal Analytics
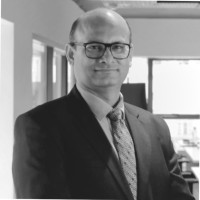
Madhav Bissa
Program Director, CoE for DSAI, Nasscom
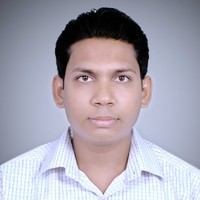
Manish Kesarwani
Advisory Research Scientist, IBM Research
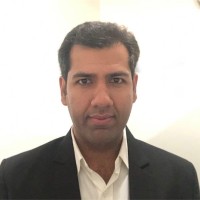
Mitesh Kapadia
Associate Director, Analytics and Data Science, Deloitte
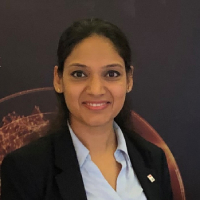
Payal Agarwal
Partner, Deloitte
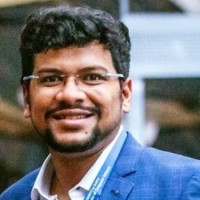
Pranay Lohia
Senior ML Researcher, Microsoft
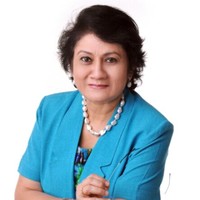
Rohini Srivathsa
National Technology Officer, Microsoft India
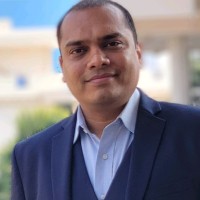
Sagar Shah
Client Partner, Fractal Analytics
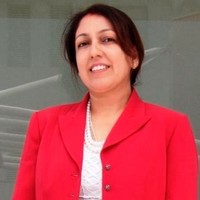
Sai Kavitha KrishnaIyengar
Director, Support Engineering, Microsoft
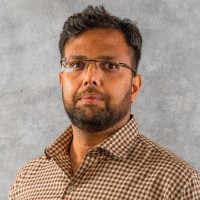
Sameep Mehta
Distinguished Engineer, AI and Hybrid Data, IBM Research
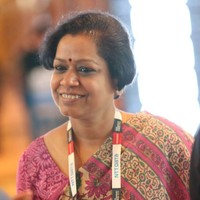
Sangeeta Gupta
Senior Vice President, Nasscom
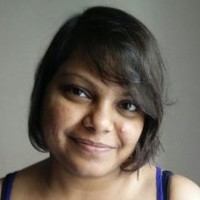
Shweta Gupta
Engineering Lead for Data and Analytics, Asia, Microsoft
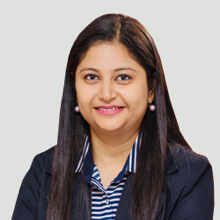
Supriya Samuel
Branding & Marketing Manager, Nasscom
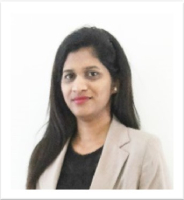
Swapna Choudhury
Associate Director Deloitte India
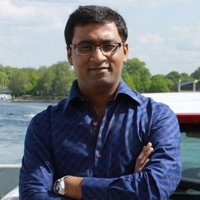
Tarun Kumar
Data Strategist, Nasscom
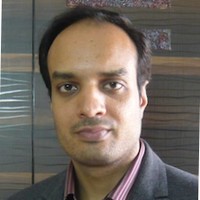
Vijay Arya
Senior Researcher, IBM Research
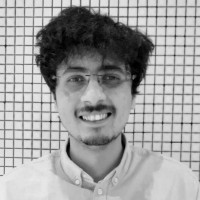
Raj Shekhar
Responsible AI Lead, Nasscom